Experimentation Deep Dive: How to Formulate a Research-Based Hypothesis

Website experimentation is a powerful tool that can help your business drive more conversions and revenue. However, it’s important not to get caught up in making your website more flashy or trying the cool things your competitors are doing. Experimentation should focus on finding a solution to a problem so you can optimize the customer experience and make better business decisions based on your learnings. In order to do these things, you need a strong, research-backed hypothesis for each and every experiment you run. So, where do you start?
Let’s take a look at what hypothesis generation is and the data sources you can use to help inform your hypotheses.
What Is Hypothesis Generation?
Generating hypotheses means defining your problem statement, inputs, and expected outcomes for your experiments based on data. The goal of hypothesis generation isn’t to create more “winning” tests but to provide more learnings for your organization. Whether your test confirms or refutes your initial hypothesis, you will gain a better understanding of your website visitors and have more data to inform future hypotheses.
Organizations in the earlier stages of experimentation maturity will likely generate hypotheses based on assumptions rather than data or first-party research. In order to remove assumptions from your tests and create experiences that resonate with your audience, it’s critical to base your hypotheses on former test results and various other data sources (e.g. analytics, heatmapping, UX research, etc).
Data Sources to Inform Your Hypotheses
No matter the size of your organization, you likely have a lot more data than you realize that can be leveraged for hypothesis generation. For example, you can review customer support or chatbot logs, listen to Gong sales call recordings, or even sit down with the customer support and sales teams to ask about the biggest pain points for existing customers and opportunities. You can also set up and distribute small surveys to your prospects (asking about things like customer motivation, their preferred buying process, and their perception of your brand/product) and leverage these responses in your test ideation and hypothesis generation.
Of course, be sure to leverage both qualitative (e.g. chatbot logs, survey responses) and quantitative (e.g. analytics) data sources and then aggregate this data to formulate your hypotheses. When possible, work to secure leadership buy-in for your experimentation program so you can invest in more in-depth research and analytics tools.
When you have the right resources, of course, you’ll want to collect advanced data (e.g. micro and macro conversions) to better identify problems and understand user behavior. Consider leveraging tools like heatmapping, session recording, and user testing which can help paint a clear picture of exactly how visitors are navigating your site and where they might be encountering friction. The more data sources you can corroborate, the better your hypotheses and tests will be.
To learn more about hypothesis generation and how you can improve the maturity of your experimentation program, download our Achieving Experimentation Maturity eBook.
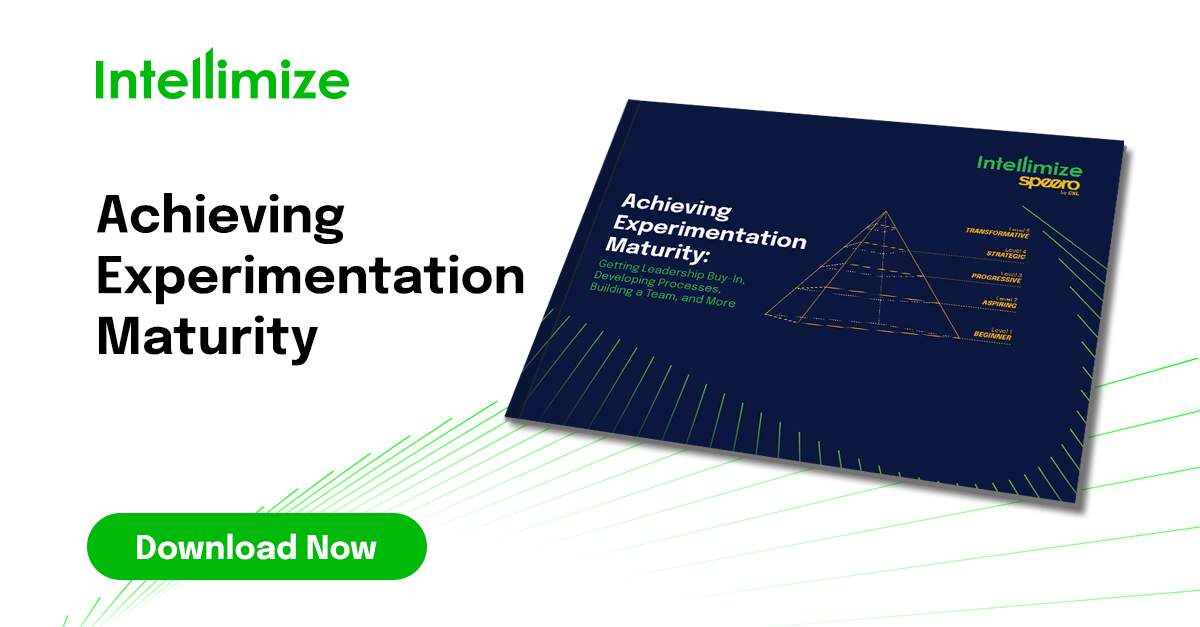