
What Is a Type 1 Error?
In statistics, a type 1 error occurs when an experiment rejects a null hypothesis. A type 1 error happens when a researcher incorrectly concludes that a positive result from testing is the true result and makes future decisions based on this erroneous proof. Type 1 errors are commonly referred to as “false positives.” They can be contrasted against type 2 errors (inaccurate acceptance of the null hypothesis), which are commonly referred to as "false negatives."
In an experiment, the null hypothesis is a hypothesis that predicts no significant difference between two populations, and that any differences that do occur are the result of an error in sampling or the experiment as a whole. Therefore, a type 1 error indicates that variations among populations made no significant difference in the study’s outcome.
How Does a Type 1 Error Differ from a Type 2 Error?
A type 1 error indicates a false positive has occurred while a type 2 error implies the existence of a false negative.
In the case of a type 2 error, a marketer may incorrectly conclude that there is no statistically significant difference between variations of a web page in terms of conversions, for example, when in reality, there is a difference. But with a type 1 error, the reality shows that there is no difference between two variations while the experiment shows that there is a statistically significant difference between them.
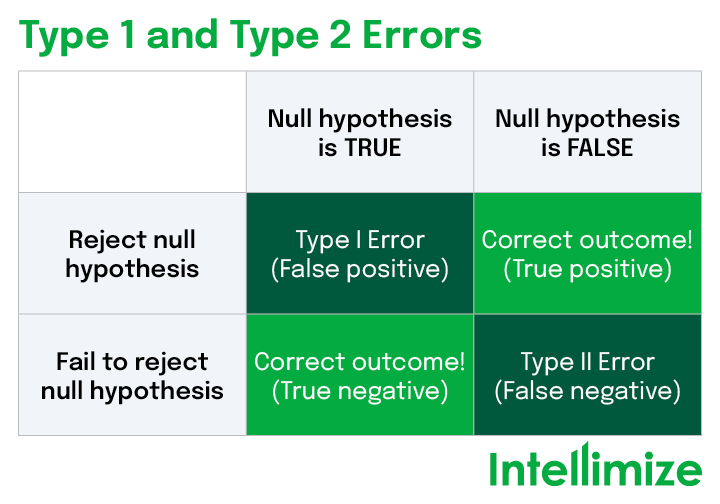
Examples of Type 1 Errors
In the world of digital marketing, type 1 errors might occur during a period of A/B testing. For example, at the beginning of an experiment, a member of the Marketing team may predict that formal email copy as part of a monthly newsletter blast will generate more CTA clicks to the organization’s website. A second email variation is created that uses more casual, conversational copy, which, per the experimenter’s hypothesis, is expected to resonate poorly with recipients and result in fewer clicks to the website. The entire experiment is set to run for a total of six months.
At the end of the first month of the experiment, the data show that more email recipients clicked the CTA in the email with the more formal copy as opposed to the casual, conversational email, with a confidence level of 90%. Based on these results, the marketer might prematurely conclude that a formal tone and voice should be used in all email marketing going forward.
But the experiment continues for the following five months. In those five months, the behavior of the recipients starts to shift, and clicks from the casual emails start to increase. Calculating the results at the end of the experiment showed no effect between the formal and casual headlines. Despite the first month’s results showing a high confidence level of 90%, premature analysis of the results produced a type 1 error, or rejection of the null hypothesis.
You may have reached this early conclusion with a sample size that wasn’t large enough to reach statistical significance, or you may have chosen criteria for variants that cause the experiment to lean in the direction that rejects the null hypothesis and automatically accepts the desired variant. These untimely conclusions or miscalculations of criteria are two ways type 1 errors can appear in A/B testing.
How Do Type 1 Errors Apply to Website Optimization and Testing?
If an experiment contains a type 1 error, resulting in a false positive, and you use this information to make changes to your website optimization strategy, this may create costly consequences for your organization. A false assumption concerning user behavior might stall and even decrease your website’s conversion rates, so removing the possibility of type 1 errors in marketing and A/B testing is crucial to ending up with accurate results.
Type 1 errors can occur for one of two reasons: random chance or poor research modalities, which are explained below.
- Despite the best efforts of researchers to make a sample as random as possible, there is no guarantee that a random sample will accurately represent the intended audience beyond the experiment. Therefore, there is the risk that the experiment’s results will not reflect the actual outcome of the chosen action when the action is applied to real life. In other words, the conclusions of the experiment may only be due to random chance.
- A/B tests require sufficient data and an adequate amount of time to reach statistical significance. If an experiment is terminated prematurely because a desired result has been reached, despite the amount of data collected, this can increase the risk for type 1 errors. That’s why it’s important for researchers to follow experiments through to completion, regardless of the direction of the data.
How to Avoid Type 1 Errors
Errors are common in statistical testing and are almost to be expected. Hypotheses by nature are never certain and are meant to be tested to determine an outcome. Therefore, testing always comes with the risk of reaching a false result, either positive or negative, so avoiding such a result can be difficult.
To prevent false positives from occurring, consider following these tips when setting up an experiment.
- Determine a set sample size. Without a set sample size, testing can result in a type 1 error, or false positive. Creating a set sample size before your experiment can lead to more statistically significant results.
- Increase your experiment’s lifetime. The longer an experiment is set to run, the more data you’ll be able to collect and, therefore, the more accurate the results are likely to be.
- Increase the statistical significance. In addition to running a longer experiment, consider increasing your confidence interval to 95% or higher, which will result in a significance level of 5% or less. To reach a higher statistical significance, you’ll also need a larger sample size.
- Confirm the accuracy of your data. Ensure the data and variants you want to test are as accurate as possible. When you have accurate inputs, you’re more likely to achieve accurate outputs.
Fortunately, Intellimize automatically includes these practices into its statistical significance calculations for A/B tests, reducing the amount of work required to be confident in your results. It’s another way we make it easier than ever to experiment effectively.
Recommended content: