
What is a Type 2 Error?
When testing hypotheses, a type 2 error occurs when the result is a false negative, or an “error of omission.” Type 2 errors do not reject the null hypothesis, which is the hypothesis that states that no difference exists between tested populations. In other words, type 2 errors lead to belief that no difference exists between tested populations when a difference actually exists.
Type 2 errors can be harmful to experiments because they can lead researchers to believe that a false finding is indeed true.
While you're unlikely to completely avoid errors, activities to mitigate them should be baked into your vetting process when designing, building, and conducting QA on tests. Let's dive into how type 2 errors differ from type 1 errors, and steps you can take to reduce them.
What Is the Difference between Type 1 and Type 2 Errors?
As mentioned, statistical testing errors can be categorized as type 1 or type 2 errors. In short, type 1 errors are false positives that lead to a rejection of the null hypothesis, whereas type 2 errors occur in the case of a false negative when the experimenter incorrectly accepts the null hypothesis even though there is a difference between the control and the variable.
Diving deeper into the different types, type 1 errors, known as "false positives," show a difference between tested populations when, in reality, there was no difference between the control and the variation.
The most common way to encounter a type 1 error is low statistical significance. For example, a stat sig threshold of 80% means there's a 20% probability of a type 1 error for each test.
Type 1 errors can also occur from external factors' impact on the test. For example, running a test during a period of extreme seasonality and assuming the results will hold true for your standard website traffic.
While raising your statistical threshold to the industry standard of 95% will help, there's still a 5% chance of encountering type 1 errors. This is a widely-accepted level of risk, the tradeoff of greater velocity outweighing the slim chance of type 1 errors.
In contrast, a type 2 error is known as a false negative, wherein there is a difference between the control group and the variation. Type 2 errors can seriously impact your experiment by furnishing results that may lead your experimentation program astray.
A type 2 error can occur when the sample size isn’t big enough or when the testing period isn’t long enough.
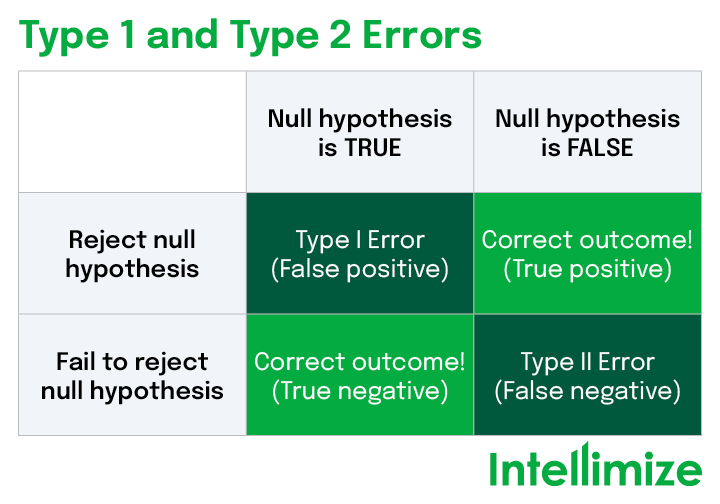
What Are Some Examples of Type 2 Errors?
Type 2 errors are essentially results that should have been rejected but that occur erroneously for myriad reasons. There are many examples of this in website optimization.
For instance, an eCommerce website may try a variation that focuses on bringing social proof above-the-fold to ensure all visitors see it, with the ultimate goal of driving more purchases. The test is activated and after two weeks the CRO practitioner peeks at the result and sees that there is no delta in the number of purchases between the variation and control, and concludes the test unable to reject the null hypothesis.
Suppose the practitioner isn't happy with this result and decides to re-run the test, but this time runs the test for a longer duration to collect more data. The second time they run the experiment they see there is a large delta between the variation and control in their effectiveness to drive more purchases.
The first time they ran the experiment they encountered a type 2 error by ending the test prematurely.
Let’s explore the effect of type 2 errors when testing a website’s ability to create conversions.
How Do Type 2 Errors Apply to Website Optimization and Testing?
In the world of A/B testing and website optimization, all types of errors should be considered, but type 2 errors are especially consequential to efficient website testing.
If a type 2 error occurs, there may have been a missed opportunity to notice a difference between two different variables. While this may seem less dangerous than the alternative (i.e., a type 1 error, or a false positive), it can still have ramifications on the performance of your website and, therefore, your site’s capability for effective conversion.
For example, you may have wanted to test two versions of landing page copy to see which resonated more with visitors and ultimately led them to request a demo of your product. In the case of a type 2 error, testing would reveal no clear statistical difference between the two copy versions, so you could simply choose the one you prefer. Avoiding this error, however, could provide valuable insight into which copy is more informative, more engaging, and results in more action on the part of the visitor.
Such an incorrect rejection of an idea, a copy change, or a visual element can hinder the opportunity for a business to succeed, simply by mistakenly believing the result of a type 2 error. If a type 2 error occurs when conducting website testing, you’ll want to look for ways to change the testing parameters to increase the power of a given test and hopefully get to a larger difference in conversion rates at the end of the test.
How to Avoid Type 2 Errors
When it comes to website testing and optimization, a type 2 error isn’t always avoidable, but there are best practices one can put into place to mitigate the risks.
- Use a large enough sample size. Depending on the results of your initial test, you may need to expand your sample size to ensure adequate data is collected. The larger your sample size, the less likely you are to experience a type 2 error. Instead of testing for longer periods of time, try testing across multiple pages to increase sample size quicker. Be mindful, however, that increasing your sample size may increase your chances of experiencing a type 1 error.
- Expand the range of variables. Making drastic changes to your variations can provide better insight and enable you to reach statistical significance and high statistical power. The higher the statistical power—or the higher the likelihood of the test detecting a real effect—the better chance you have of avoiding a type 2 error.
When you start building out your hypothesis experiment, it’s important to consider the possibility of a type 2 error and design the experiment to try to avoid it.
Recommended content